Data Management in Farming: Discovering the Opportunities
At this age, data is crucial in every industry, and agriculture is not an exception. According to Statista, the smart (digital) agriculture market is expected to grow from around $15 billion in 2022 to $33 billion by 2027.
But how you get the data, and what you do with it matters more than you’d think. Poor data quality, security breaches, or badly optimized agriculture data management can lead to millions in losses.
Now, we will talk about the importance of data management for farmers, how you can set up a system to collect and analyze data for your business, and what challenges you will face along the way.
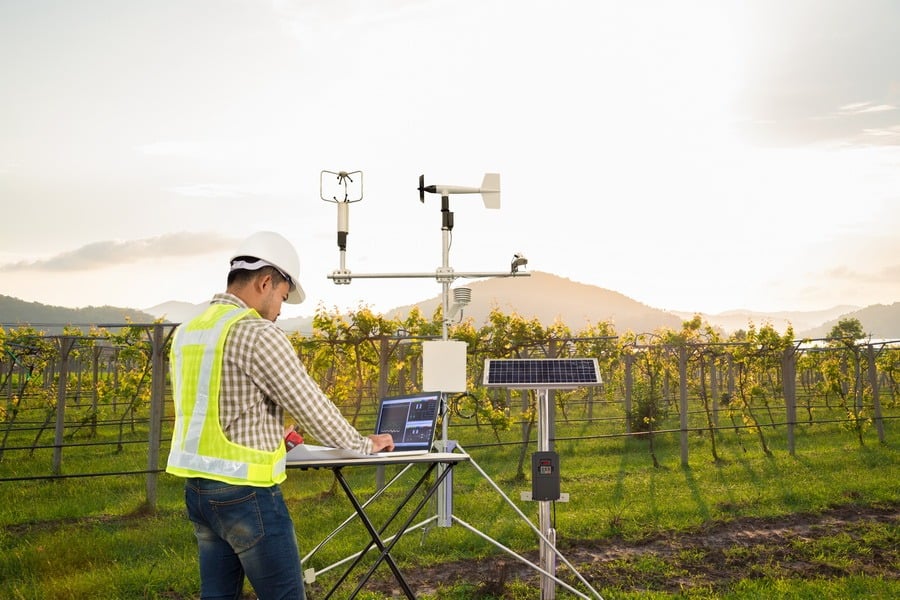
Data management allows farmers’ organizations and agribusinesses to make informed decisions.
If you use it well, data can tell you how to reduce water waste, improve farm productivity, enhance crop yields, or map out your field.
Effective farm data management serves as a base for precision agriculture, which is the modern approach to optimizing farm operations.
It drives sustainability and increases ROI by reducing the waste of resources, and applying them precisely as needed.
But let’s dive into the details of how you can set up data management on the farm, and how you can solve the issues you’ll encounter.
Create a data-driven strategy for your agribusiness
Integrate custom-built data management and AgTech solutions to maximize your ROI.
DISCOVER OUR SERVICES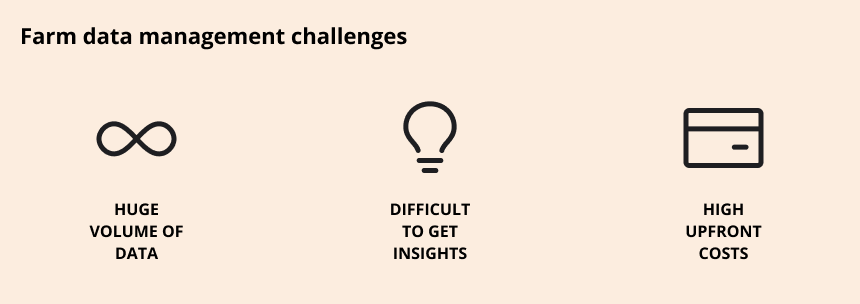
While data management can refer to handling any amount of data, data management for agriculture usually falls into the big data category.
Here are the top 3 challenges you’ll have to solve.
1. Huge volume from various sources, in various formats
Data management for farmers is difficult because you can collect data from many different sources: satellites, sensors, etc. Managing and safely storing such a large dataset requires adequate infrastructure.
2. Difficult to get insights
Handling big data requires experts. If you don’t have your own tech team, it is a good idea to acquire a tech partner. To avoid errors, you need to set up the system correctly and automate the entire process.
3. High upfront costs
Setting up custom-built high-tech systems to collect and manage data is costly. So it might only be worth investing in such technology if you have large farms with large-scale operations.
It is hard to estimate the ROI in the long run, but some estimates suggest that the cost of precision agriculture would return within 2 years.
Next, we’ll cover the process of farm data management – along with the solutions to these problems.
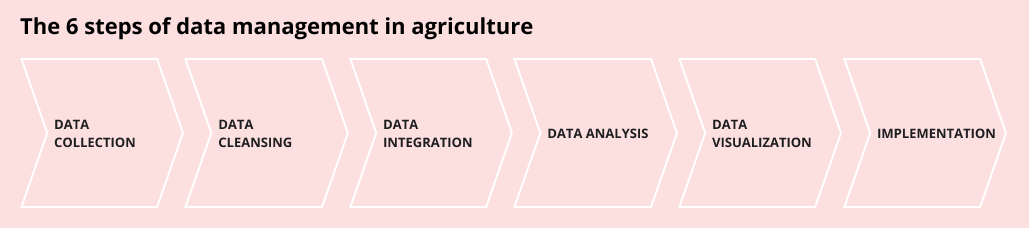
1. Data collection
Gathering the necessary data is the first step in this process. Farmers and other decision-makers need information about the weather, the soil, the irrigation systems, etc.
Common technologies used for collecting data are:
- In-field sensors and IoT tools to learn about the nutrition and moisture levels of the soil
- GPS technology to gather location-based data with high accuracy
- Satellite-based technology for weather-related information
- Smart farm machinery to learn about the quality and quantity of the crops
2. Data cleansing
Your insights are only as good as your data. A Gartner survey showed that organizations lose $15 million/year on average due to poor data quality.
So to ensure that your data is up-to-date, correct, and relevant, some ‘data scrubbing’ is necessary.
During this process, you remove any information from your dataset that could lead to errors or misinterpretation.
Data transformation is also part of this step usually because it’s crucial to convert the unstructured data into a format your tools can work with.
When it comes to pre-processing technologies, we have to mention ETL (Extract, Transform, and Load) tools. These are important for cleaning, integrating, and transforming the heterogeneous raw data with high precision.
Farm data management and cleaning use case
A real-life example is the yield data cleaning and calibration service offered by GeoPard Agriculture. They create datasets about the yield based on satellite imagery and sensors.
Then they use algorithms and models to improve the data quality, and by doing so, improve crop yields as well by 10-12%.
3. Data integration
When your separate datasets (from different sources) are ready, it’s time to combine them. Let’s say for example, that you have data both from satellites and sensors.
Then storing and managing data is the next step, so you need to integrate these sources with your data storage. Cloud-based storage is the most popular because it is scalable, flexible, accessible from anywhere, and cost-efficient.
And since you’re dealing with a large amount of data, it is important to have simplified maintenance and reliable support.
Besides cloud computing, we have to mention fog and edge computing as well.
Edge computing is used for local data storage and analytics. This technology is very useful because most of the data is gathered in the field and needs to be processed on-site. This results in quick decision-making and actions. Such as watering the crops immediately when needed.
Fog computing supports edge computing. It also helps with storing and managing a large amount of data, but instead of processing it closer to the data source (like at the sensors or IoT devices), it distributes the data among a network of devices.
In a sense, fog stands between cloud and edge computing.
- Edge supports real-time data analysis, and immediate decision-making.
- Fog also supports real-time data analysis, but it can decide between processing the data locally, or sending it to the cloud.
- Cloud provides a permanent storage, and handles the less time-sensitive data processing.
To manage your data effectively, you might need to use all of these technologies. Worry not, if you’re uncertain about this topic, all you need to do is contact us to gain clarity.
Security is also a crucial factor in choosing your data storage. According to IBM, the global average cost of a data breach in 2023 was $4.45 million. Data in the agriculture sector is highly valuable, both for the competition and for hackers.
That’s why data encryption, controlling data access within the organization, and constant auditions are necessary.
4. Data analysis
Some would say that we’ve arrived at the most important step. Based on your business goals and the type and volume of data you have, you need to decide on the type of insights you need.
There are 4 types of data analysis – and 4 questions you can seek the answers for:
- Descriptive analytics (What happened?)
- Diagnostic analytics (Why did this happen?)
- Predictive analytics (What might happen in the future?)
- Prescriptive analytics (What should you do next?)
To have accurate insights, you might also need to combine your current information with historical data.
Then you can choose the right data analytics techniques and tools. For real-time data analysis you need different tools than for recognizing trends. The most innovative tools all use artificial intelligence (AI) and machine learning technologies in some form.
For example, predictive analytics tools use a variety of methods, including modeling and machine learning to seek data patterns, and create forecasts that are crucial in agriculture.
5. Data visualization
Humans are inherently visual creatures because our brain processes images much faster than text. So to make sense of the numbers, you need to turn them into diagrams, charts, or maps.
Data visualization allows you to recognize patterns and connections, and make predictions and data-driven decisions.
Agriculture data management use case
In many cases, AgTech companies create solutions that combine all these steps.
For example, our long-term client, Origin Digital is developing smart farming tools with our help.
These tools can handle every part of the process: from gathering data (from satellites and other sources) to visualizing the insights on clear field maps. Farmers can have access to these insights from the comfort of their own phones if they wish, thanks to the custom application.
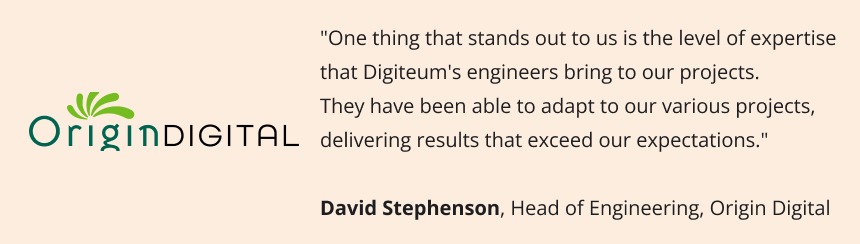
6. Implementation
The final phase of implementing data management is to execute the actions you determined based on the insights.
For example, if your data shows a lack of nutrients at a certain part of your field, you can apply the exact amount needed on the spot.
This entire process can be automated. Just think about automated sprinklers or other automated field systems that perform actions based on the data input.
In many cases, all this information is available on dashboards in portals or client applications, so the decision-makers have easy access to all the insights in one place.
Based on the tech partner you choose, your custom software can be tailored to your company’s specific needs.
Imagine: having access to all that data and your farm digital strategy at your fingertips!
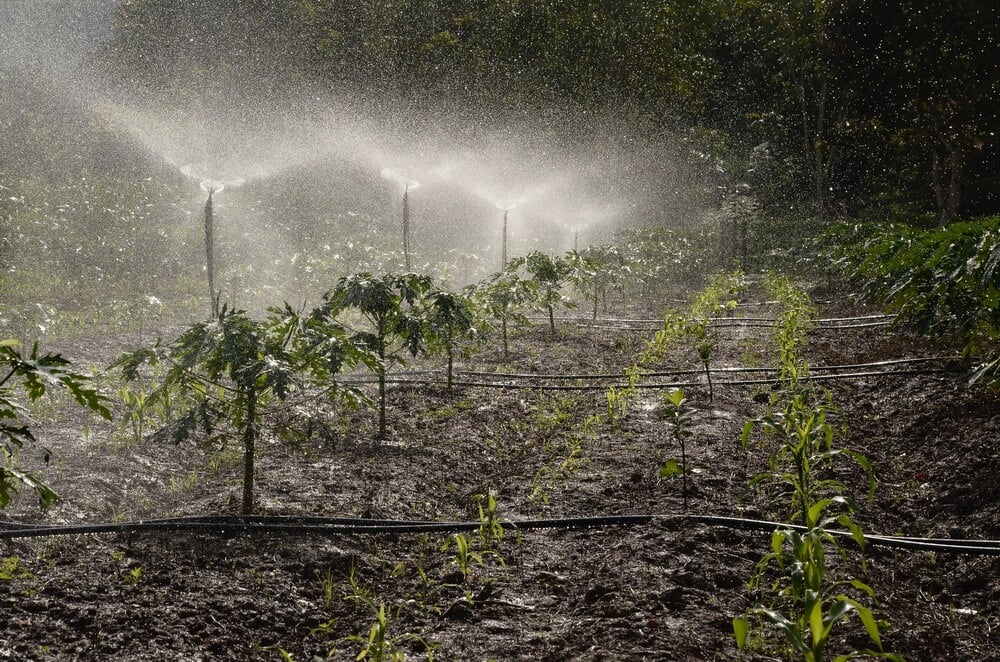
Our decade-long experience in providing agriculture software development services proves that you would greatly benefit from our cooperation.
We have extensive knowledge in data engineering, and have worked with multiple clients in the agriculture industry.
We can support you with:
- Creating effective data pipelines to gather, process, and manage data and deliver insights to decision-makers
- Integrate data analytics and visualization tools with your existing system to make sense of the data
- Incorrect data. Errors, such as duplicate, missing, or outdated data, can lead to incorrect insights and bad business decisions.
- Develop custom intuitive interfaces and dashboards to cut the time to insight, and accelerate the decision-making process
- Augment your own engineering and data teams to help cope with large volume of data management and processing
And what you will always receive is:
- a fast project kick-off,
- a business-oriented approach,
- and proactive communication.
Check out the case study below for more details on how could help you hit your goals.
Custom data management system for an agriculture company
Our client, an agriculture analytics and research company was facing serious issues with document management. They had documents in several different formats: both on paper and in non-standardized PDFs.
To help them optimize their documentation process, we’ve developed a custom web application. The goal was to automate the processing of invoices and other documents and to extract all important information without losing much data.
This project involved:
- A custom web system for automated text recognition that allowed them to process PDFs in multiple formats, even of various quality
- The use of cloud services to store the large amounts of data securely
- Custom data extraction algorithms for high accuracy
The cooperation was a success: the new system can extract data with up to 80% accuracy. There’s an opportunity to scale and improve it in the future, so this percentage can go higher. The automation freed up human resources for our client and helped them avoid costly errors.
Read more about this success story…
If you’d like to achieve similar results, check out our big data management services, or contact us below.
Increase your ROI with data-driven decisions
Avoid ‘flying blind’, manage your data with our help!
HIRE EXPERTSConclusion
Digital agriculture is a crucial part of the food supply chain. To feed the growing population sustainably, we need to implement new, innovative ways to optimize processes and increase the yield.
It is time for you to embrace this new era as well, and we’re here to help you every step of the way.